What is Generative AI? How is it helping change the World?

Author : Ben Kumar 7th Jul 2023
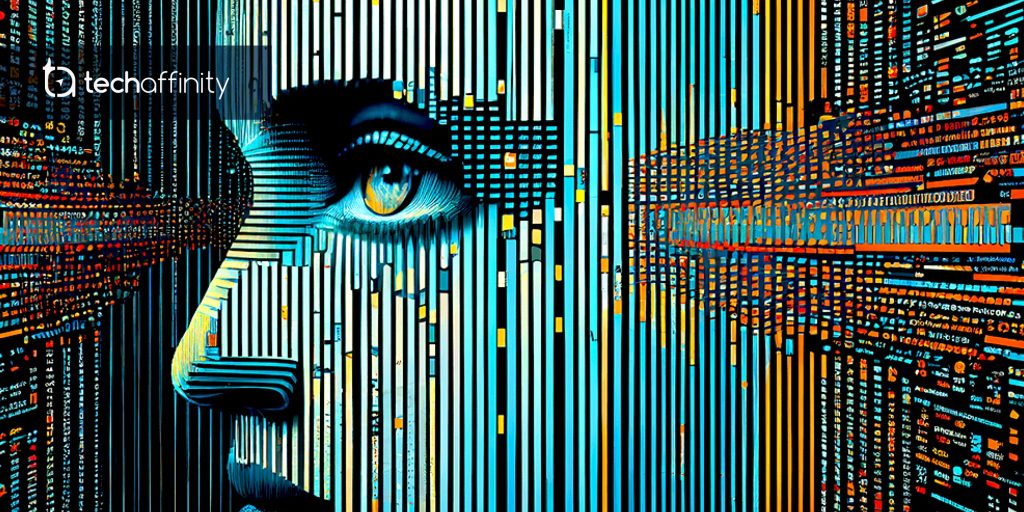
In recent years or maybe months for some, this groundbreaking technology has captured the imagination of researchers, scientists, and creatives alike, as it holds the potential to transform the way we interact with and create content. From generating lifelike images to composing music and even crafting engaging stories, Generative AI has emerged as a powerful tool that pushes the boundaries of what’s possible in the realm of artificial intelligence. In this article we will explore the applications, advancements, implications and use of Generative AI, and uncover the ways it is shaping various industries, sparking innovation, and redefining the very concept of creativity.
What is Generative AI?
Generative Artificial Intelligence (AI) is a field of AI that focuses on creating new and original content, such as images, music, or text, using machine learning models. These algorithms are trained on large datasets to learn patterns and then generate outputs based on that training. Generative AI differs from traditional AI models that focus on prediction by aiming to generate novel and unique content. Techniques commonly used in generative AI include deep learning, neural networks, and reinforcement learning. The applications of generative AI range from image generation to text generation, music composition, and video synthesis.
Generative AI in scientific terms
Generative Artificial Intelligence (GAI) is a field that focuses on creating open-ended systems with non-linear properties to develop intelligent machines. GAI utilizes post-structuralist theories of possibility spaces and neo-cybernetic mechanisms like feedback to actively manipulate the phase space of possibilities.
It combines both bottom-up and top-down approaches, using bottom-up procedures to generate possibility spaces and top-down methods to sort out the structures that are functioning the worst. The goal of GAI is to create open-ended systems that have interesting properties for the scaffolding of an artificial mind.
GAI sidesteps the traditional argumentation of top-down versus bottom-up by incorporating both mechanisms and involving the environment or humans in steering the development processes.
What are some generative AI examples?
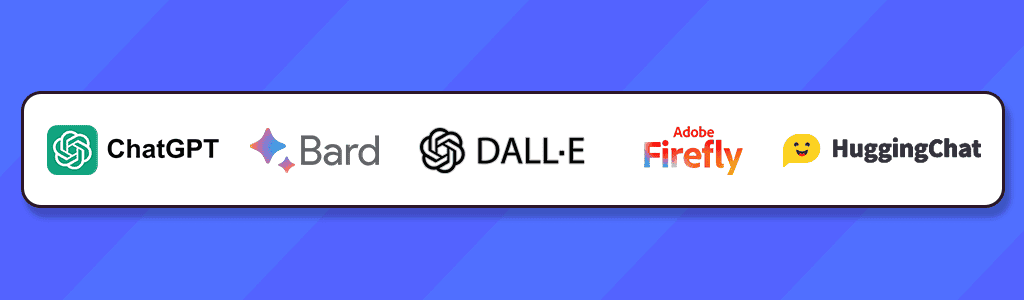
Generative AI capabilities is a rapidly growing field with many examples across various industries with tools like ChatGPT, FireFly by Adobe and many more. Here are some examples of generative AI:
ChatGPT: An AI chatbot, ChatGPT by OpenAI is a conversational chatbot that uses generative AI to produce new content, including computer code, essays, emails, social media captions, images, poems, excel formulas, and more within seconds.
DALL-E: DALL-E is a generative AI technology developed by OpenAI that enables users to create new images with text to graphics prompts.
Google Bard: Like ChatGPT, Google Bard is an AI-powered chatbot tool developed by Google that uses natural language processing and machine learning to simulate human conversations. It is designed to provide realistic, natural language responses to user questions and can be integrated into websites, messaging platforms, or applications.
MusicLM: Google’s unreleased AI text-to-music generator.
Adobe Firefly: Adobe’s generative AI lets you create virtually anything you can imagine, and with its new Generative Fill tool, you can non-destructively add, remove, and replace parts of an image using AI.
Variational Autoencoder: A generative AI model that can generate new financial data or conduct automated financial analysis tasks.
Charisma AI, Hidden Door, Unity Machine Learning Agents, Houdini: Examples of generative AI applications use cases across industries.
Image and video generation: Generative AI can be used to create realistic images and videos based on specific settings, subjects, styles, or location.
Text generation: Generative Artificial Intelligence can generate coherent and contextually relevant responses to prompts.
Data generation: Generative AI models to create data and insights for business activities.
Generative Artificial Intelligence has revolutionized the way we convert text into images and create lifelike visuals with customized settings, subjects, styles, or locations. It enables users to effortlessly produce visual content for a wide range of applications, including media, design, advertising, marketing, and education. This evolving technology holds immense potential for businesses to explore novel avenues of innovation and propel advancements within their specific fields.
What are the differences between Generative AI vs Machine Learning?

Generative AI
- Focuses on creating new and original content
- Uses algorithms to generate new data, such as images, videos, audio, text, and other types of content
- Can both learn to generate data and refine its outputs
- Is a subset of deep learning
- Has applications in various industries, including gaming, marketing, customer service, and creative arts
- Raises concerns about legal, ethical, political, ecological, social, and economic issues
Machine Learning
- Emphasizes learning from data and making predictions
- Uses algorithms to identify patterns within a training data set
- Can be used for predictive analytics, natural language processing, image recognition, and other applications
- Is a powerful sub-category of artificial intelligence
- Can be used to address complex challenges
- Can be used in tandem with generative AI
Generative AI in Healthcare

AI-powered risk assessment tools are used in the field of mental health to predict successful antidepressant medication, characterize depression, predict suicide, and predict bouts of psychosis in schizophrenics. It can help in the design and exploration of nucleoside analogs, which are important in antiviral drug discovery and synthetic biology.
Generative AI can assist in drug discovery by generating candidate molecules with suitable absorption, distribution, metabolism, excretion, and toxicity (ADMET) properties, potentially speeding up the process of finding promising drug candidates. Generative Artificial Intelligence can support medical imaging analysis by generating synthetic images for training and augmenting datasets, improving the accuracy and efficiency of diagnostic processes .
Generative Artificial Intelligence is still in its infancy for business use, and there is limited knowledge about its potential for malicious misuse at a large scale. Generative AI models for designing drug candidates using prior biological and chemical knowledge have advantages and disadvantages that need to be considered.
What are some challenges in training generative AI models
Training generative AI models can be challenging due to several factors, including:
Bias in training data: Generative AI models may inadvertently learn and perpetuate biases present in the training data, leading to unfair or discriminatory outcomes.
Quality of generated outputs: Generative Artificial Intelligence systems may not always produce high-quality outputs, and the generated outputs may contain errors or artifacts. This can be due to a variety of factors, such as a lack of data, poor training, or an overly complex model.
Control over generated outputs: Generative Artificial Intelligence models may produce outputs that are difficult to control or modify, making it challenging to ensure that the generated content meets specific requirements.
Integration with legacy systems and data: One of the biggest concerns when implementing generative AI is integration with legacy systems and data. These older systems are often difficult to change and integrate, making the adoption of new AI technologies seem like an insurmountable task.
Data privacy and security: Generative Artificial Intelligence models require large amounts of data to train, which can raise concerns about data privacy and security.
Scaling and integration: Scaling and integrating generative AI systems into existing workflows and processes can be challenging, requiring significant resources and expertise.
Talent acquisition and retention: Companies may struggle to find and retain talent with the necessary skills and expertise to develop and maintain generative AI models.
Intellectual property issues: Generative AI technology can collide with intellectual property issues, leading to copyright issues and other legal concerns.
How is Generative AI used in different industries?

Generative Artificial Intelligence has many applications in various industries, including marketing, gaming, art, finance, and healthcare. Here are some examples of how generative AI is used in each industry:
Marketing
Generative AI can be used to automate content creation, data analysis, personalized marketing, and enhancing customer experience. It can create content from scratch, such as text, images, and videos, and help marketers maintain a consistent, high-quality content stream. Generative Artificial Intelligence can also optimize marketing strategies through A/B testing of various elements, leveraging predictive analytics and data-driven recommendations to ensure maximum return on investment.
Gaming
Generative AI can be used to create fresh and unique content for gamers, such as potions, weapons, and characters. It can save valuable time and resources by creating high-quality images in a matter of hours that would otherwise take weeks to generate by hand. Generative AI can also create an endless number of variations in a highly iterative way, providing game developers with a wealth of creative opportunities and inspiration.
Art
Generative AI can be used to create high-quality artistic media for visual arts, concept art, music, and literature. It can generate one-of-a-kind pieces of art in just a few clicks, making it an exciting tool for artists, designers, and enthusiasts alike. Generative AI can also help artists and designers explore the vast potential of AI and push the boundaries of what’s possible in art.
Finance
Generative AI can be used to process, summarize, and extract valuable information from large volumes of financial documents, such as annual reports, financial statements, and earnings calls, facilitating more efficient analysis and decision-making. It can also make predictive analytics about future trends, asset prices, and economic indicators by learning from historical financial data. Generative AI can also generate customized financial reports or visualizations. Additionally, generative AI can be used to improve risk management, enhance fraud detection, personalize services, and refine investment strategies.
Healthcare
Generative AI offers numerous possibilities in the field of healthcare, spanning areas such as drug exploration, disease detection, patient treatment, medical imaging, and scientific investigations. It can analyze vast amounts of data and recognize patterns, providing doctors and healthcare providers with valuable insights into patient care. Generative AI can also contribute to identifying fraudulent activities and detecting anomalies in financial transactions. Additionally, generative AI can transform the future of healthcare by augmenting human creativity and productivity, improving patient care, and lowering costs.
Fashion
Generative AI is being used in the fashion industry in various ways, including product innovation, marketing, sales, and customer experience. One of the main applications of generative AI in fashion is mass customization, which involves creating something for a larger audience but customizing it to suit individuals’ needs or desires. Generative AI can also be used to create fresh and unique content for fashion designers, such as sketches, patterns, and designs. Additionally, generative AI can be used to process and analyze large volumes of data, such as customer preferences and trends, to help fashion businesses become more productive, get to market faster, and serve customers better.
It can also be used to create virtual try-on experiences, allowing customers to see how clothes look on real models with different body shapes and sizes. This can help customers make more informed purchasing decisions and reduce the number of returns.
Another application of generative AI in fashion is trend forecasting. Generative AI can analyze vast amounts of data and recognize patterns, providing fashion businesses with valuable insights into upcoming trends and customer preferences. This can help fashion businesses stay ahead of the curve and innovate their fashion game.
Frequently Asked Questions (FAQ) about Generative AI
Generative AI is a type of artificial intelligence that can produce various types of content, including text, imagery, audio, and synthetic data. It relies heavily on Transfer Learning and Large Language Models (LLMs) to understand context and generate new data.
Generative AI uses deep learning models to generate digital content from natural language descriptions, called “prompts”. The models are trained on large datasets of existing content and use algorithms to identify patterns and structures within the data to generate new and original content. For example, a generative AI model can take a text prompt and generate a photorealistic image or a piece of music.
The most popular generative model in Language Generation (LG) is the GPT (Generative Pre-trained Transformer) model. Large tech companies such as Google, Microsoft, and OpenAI are investing heavily in generative AI research and development.
Generative AI’s language understanding has limits, and it is sometimes unable to distinguish between similar prompts. It can also generate inappropriate content, such as pornographic or violent content.
Generative AI has many applications in various industries, including advertising, marketing, design, and art. It can be used to create custom content for websites, social media accounts, and other online platforms, helping businesses and individuals save time and resources while maintaining a consistent, high-quality content stream. It can also be used to improve the quality of outdated or low-quality learning materials, such as historical documents, photographs, and films.
Yes, generative AI can create various types of content, including text, imagery, audio, and synthetic data.
The quality of generated content can be evaluated by comparing it to existing content and assessing its accuracy, coherence, and relevance to the prompt.
To mitigate the risks associated with generative AI, it is important to carefully monitor and regulate its use, ensure that it is used ethically and responsibly, and implement safeguards to prevent the generation of inappropriate content.
